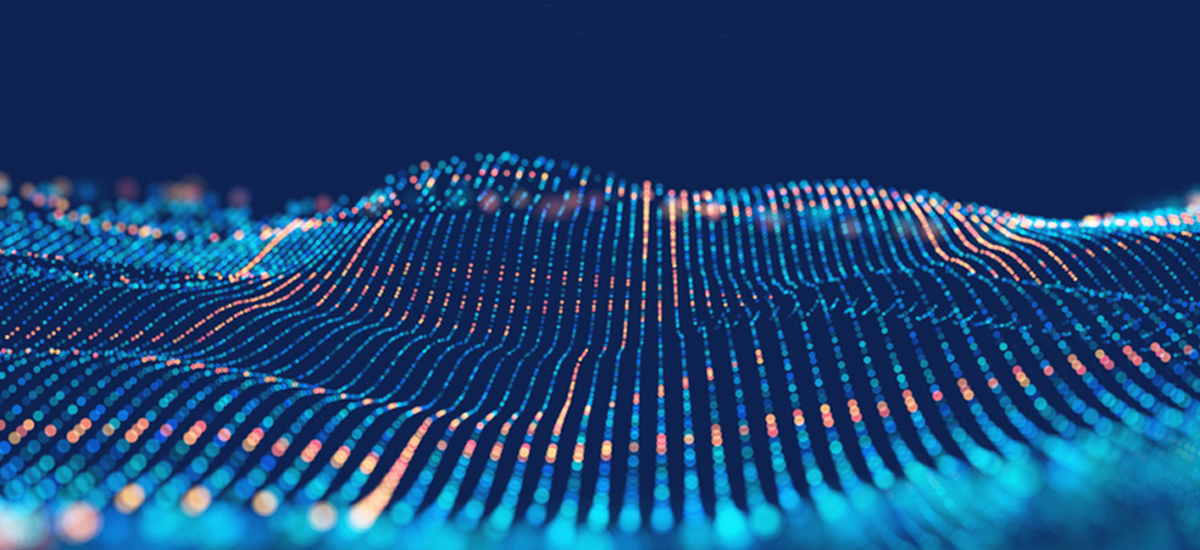
Listen to this blog
Do you Know What is Essential to Drive the Most Successful Business Decisions? Data Quality!
Data collected from a vast array of sources is ingested and processed by a modern data platform and made available to business users to extract valuable insights and drive business decisions. You may be wondering what role data quality plays in this process. Good quality data provides vital information, which in turn develops better knowledge and understanding, leading to superior business decision-making and enhanced customer satisfaction.
Consider the example of a courier service company: every time they receive an order, the address and other details of the shipper and the receiver are recorded by the company. The data received can sometimes lack important information, leading to postmen delivering orders to the wrong address, which reduces the credibility of the courier company. This illustrates the expression "garbage in, garbage out"; when poor-quality data is ingested for any kind of business use, it leads to inferior decision-making, which eventually results in a loss of credibility.
So, what is data quality? Think of it as a source of truth. As vast amounts of data from various sources are stored and analyzed within a modern data platform, their quality can become questionable. Good quality data is that which meets the needs of business users and aligns with the exact purpose for which it was collected. Picture a hierarchical model, with data at its base. This data moves one level up to provide useful information and practical knowledge, which is then transformed to drive business decisions. Data quality determines the effectiveness of such decisions and whether they'll help businesses make accurate and informed decisions.
However, as data is gathered from a wide variety of sources and brought into a modern data platform, it can be subject to many ambiguities. There can be numerous data quality issues, such as duplicate records, missing information, incomplete records, and inconsistent formatting, which can impact the accuracy and reliability of insights. Organizations become more prone to losing credibility when decisions are based on poor-quality data, which is why it becomes imperative to proactively measure and manage it.
Measuring and Evaluating Data Quality
The quality of data can be measured and evaluated based on a few dimensions. These identify how useful the data will be when applied to specific business decisions.
These dimensions allow organizations to measure and manage the quality of data, ensuring that it is fit for its intended use.
Visionet has always emphasized the importance of data quality management and employs a few techniques to restore and strengthen it wherever possible. Data Validation and Data Profiling are two such methods that help enhance the quality of data. The former ensures that the data meets industry standards by verifying its accuracy, consistency, and completeness, while the latter helps understand the structure of the data and identifies relevant patterns and relationships.
Another method of managing data quality is Data Standardization, which helps bring various unrelated data sets to follow a particular format. Data Matching is a technique that helps identify if two or more similar kinds of data provide the same insights, and once Data Quality Reporting is aided by Data Profiling and Data Matching, it helps measure the key performance indicators for data quality. Finally, Data Quality Monitoring carries out routine quality checks, where artificial intelligence can easily identify, improve, and update any missing or wrong information so that companies can use it to their benefit.
Master Data Management
Master Data Management refers to maintaining a complete, master record, also known as the golden record, for consumers, products, employees, or any other entity. As organizations collect data from an increasing number of sources, data quality can become unreliable. Master Data Management can help organizations consolidate and deduplicate conflicting records to create a golden master record that contains the most accurate, complete, and reliable information and acts as a single source of truth. In this way, Master Data Management and Data Quality go hand-in-hand and enable business users to have access to good quality, accurate, and reliable data.
Two main examples of master data are customer master data and product master data. Customer data is collected and maintained to manage and enhance customer relationships. All relevant information on customers, such as their name, age, address, and contact number, is stored in a single master file. This acts as a single source of truth to identify the purchase history of customers, their likes and dislikes, and to pitch the right products and services to them. Customer data becomes more transparent across all channels, and the right kind of customers are targeted with the right products and services. Product master data is the single master file maintained on all products, such as their names, quantities, descriptions, prices, etc., in order to manage inventory, track sales at all product hierarchies, and keep optimal stock levels to avoid any inventory shortages. Master Data Management can also help keep a single and consistent file on all of the company’s financial data, such as its revenues, costs, profit margins, and expenses, to make more informed decisions in the future.
The set of such golden records helps companies maintain uniform and complete data on their customers and products. This way, they can follow a more strategic decision-making process with the help of complete and consistent information. Locating data becomes easier, and information becomes more accessible when stored in a single file, which makes decision-making less time-consuming and more efficient.
Significance of Data Quality
The following are some key benefits of implementing a robust data quality framework in a modern data platform:
Accurate Information: High-quality data provides the most vital and authentic information, which can help businesses take the most beneficial approach across all business areas.
Superior Decision-Making: Data available for consumption for business users should act as a single source of truth to ensure consistency across the organization and to drive effective business decisions that reap favorable outcomes.
Increased Interdepartmental Transparency: As good quality data is stored in a centralized location, it is convenient for all users within an organization to extract and use it for various data and analytics use cases. This also makes information more transparent as all departments can have access to the same information across an organization. Teams become better aligned with the decisions made, which increases their efficiency and effectiveness.
Enhanced Customer Satisfaction: As a modern data platform stores substantial amounts of data, and if this is of high quality, it may provide the most authentic insights on the wide variety of customers’ needs, wants, and major market trends. Businesses can use this information to meet the exact needs of their customers, hence maintaining and enhancing the customer base.
Reduced Costs: It can be extremely costly for organizations to make decisions based on data that is of inferior quality. Taking decisions based on poor-quality data can cause companies to lose capital and can result in a failure to meet revenue targets. A robust Data Quality framework applies data quality rules to refine data and prevent organizations from incurring these costs as well as missing out on potential revenue.
Increased Efficiency: Good quality data can easily be consolidated so that useful information is searched for and extracted for better and more efficient decision-making throughout all business domains.
Best Data Quality Practices
Companies are always striving to improve the quality of their data so that they lead to successful business outcomes and the most efficient practical results. Some best practices can also be followed to proactively improve the quality of data to meet specific needs:
⦁ Data quality management should be added as an activity to the data governance framework, which sets the policies and standards for data.
⦁ Ensure that all C-level management is also involved in driving data quality initiatives. Data analysts can easily discern any quality issues in data through interdepartmental collaborations.
⦁ Be mindful of problems caused by poor quality data and acknowledge the importance of good quality data.
⦁ Perform root cause analysis for any issue related to data quality. These issues must be proactively identified and resolved.
⦁ A log should be maintained for data quality issues that arise. When was the issue identified, what was the reason for it, what were its consequences, and how was it addressed should all be recorded.
⦁ Manual data entries should be avoided if possible, and special care must be taken for any exceptions that require manual data intervention. Data should be gathered from credible sources to support informed decision-making.
⦁ Data Quality KPIs should be established, which go hand-in-hand with the Data Quality dimensions mentioned earlier to evaluate the quality of data.
We at Visionet stress the importance of data quality and help businesses identify any roadblocks to data usability and clean data as per users’ needs. We have assisted our clients operating in varied industries to transform their data so that it fits the highest standards of quality and aids in informed decision-making.
Use Case
Refining data quality for a leading US healthcare organization has been one of our most notable ventures. We started by identifying quality issues within their patient records. As we already have extensive experience in managing data quality across modern data platforms, our SMEs used cutting-edge technology and tools to help identify any missing or incomplete records.
They made sure the data was improved to be accurate, valid, and up-to-date. Measuring and updating it based on the various dimensions of data quality helped our client maintain complete and consistent patient records to successfully base any future arrangements on. It also aided in providing a 360° view of their patients, which helped them lay out a more comprehensive representation of each patient and led to the client delivering high-quality, patient-centric healthcare. All of this also led to more accurate, rational, and effective decision-making, which supported the client in meeting their business objectives.
We have expertise in a variety of industries, including healthcare, financial services, and retail. Our portfolio includes numerous success stories of businesses that have improved their operations and decision-making by leveraging our expertise in data quality and governance. Our solutions allow you to be proactive, competitive, and innovative as you meet your business objectives. Contact us at Visionet, and let us help you on your journey towards success.