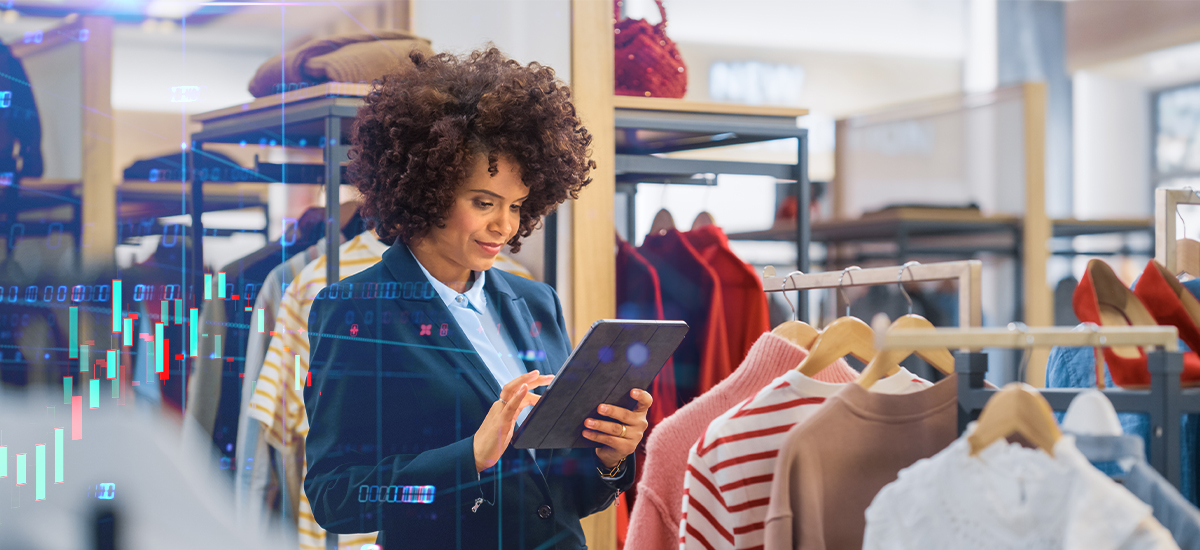
Listen to this blog
Consumer Demand is Interconnected
The use of predictive modeling to forecast future demand and sales is becoming increasingly prevalent, and many retail companies are investing in strengthening their data pipelines. Data is the key component in building a successful category forecasting projection model. As such, businesses should prioritize effective and robust methods of collecting and curating data that accurately represents their business activities.
After all, the best predictor of future demand is historic demand, right? The answer to that question is not a simple Yes or No. While internal historic data is important, it is not the only factor that can influence consumer choices. This has been particularly evident in the past couple of years with the COVID-19 pandemic, which has demonstrated that external events can have a significant impact on any retail organization, regardless of its size or location. Therefore, it is important to also consider external factors that may impact consumer behavior.
How do external factors drive retail demand?
To reliably and accurately forecast demand, retail companies need to leverage data that represents key external drivers of consumer behavior. These include, but are not limited to:
Economic Data: Economic factors such as inflation, GDP, interest rates, and unemployment rates can significantly affect retail demand. In times of economic growth, consumers have more disposable income and tend to spend more. Conversely, in times of economic recession, consumers have less disposable income and tend to spend less.
Demographic Data: Retail demand can also be influenced by demographic factors such as population size, age, income level, and gender. For instance, retailers may see a surge in demand for baby products in areas with high birth rates or increased demand for health and wellness products in areas with an aging population.
Social Data: Social factors such as trends, lifestyles, and cultural preferences can affect retail demand. For example, consumers may be more interested in purchasing environmentally friendly products or prefer to shop online rather than in-store.
Weather Data: Weather conditions can also affect retail demand. Seasonal products like clothing SKUs change demand cycles based on whether the weather is hot or cold.
Competitive Data: Retail demand can be influenced by competitive factors such as the availability of substitute products, the pricing of competitors, and the quality of products offered by competitors.
Technological Data: Technological advancements such as the rise of e-commerce, mobile shopping apps, and social media can affect retail demand. Retailers must adapt to changing technological trends to remain competitive and meet customer demand.
To stay competitive in a fast-evolving retail landscape, retailers need to be mindful of these external factors and leverage them to make informed predictions for future sales.
Identifying and Analyzing Relevant Influencers
The first and most important step in enriching your forecasts with external influencers is to start by identifying relevant data sources. While some data may be readily available, it is important to assess whether it is truly relevant to your business and the products or services you offer. For instance, while online consumer search trends may seem like a valuable addition to any retailer's data toolkit, they may not accurately reflect customer intent in categories that heavily rely on in-store purchases, such as cosmetics and lipsticks.
Identification and selection of data sources should not solely rely on intuition. Correlation analysis must be conducted to determine whether a data asset is a statistical match or can provide a lead/lag signal for predictive use.
This analysis can filter indicators by correlation strength and context, such as geography, industry, scope, and channel. Our clients are often surprised by the indicators that predict their business performance. Some indicators may be obvious, while others may be less apparent, such as wage increases before luxury goods purchases or alcohol consumption as an indicator of power tool sales. The bottom line is that each company, each brand, and each category has a unique set of leading or lagging indicators that most consistently happen before or after a change in performance.
Data Collection and Processing
Once you have identified the relevant data sources, you need to gather the data. Depending on the sources, this could involve web scraping, data mining, or purchasing data from third-party providers. It is important to ensure that the data is accurate, up-to-date, and relevant to your forecasting needs. For instance, customer ratings and reviews can be a useful indicator of consumer sentiment towards a category, but it needs to be at the taxonomy of the retailer's operations. Otherwise, that data can reflect out-of-scope products and misrepresent customer intent.
It is also essential to have access to the most up-to-date data for any predictive models to deliver information at the highest level of accuracy, and in turn, identify shifts before they happen. Many companies still rely on annual reports or one-time data inputs. In current conditions, those reports and data can become outdated in less than three months. As such, you need a systematic process for collecting external data that is designed to update your assets in real-time. Business executives will have access to the most recent information available if signals change or conditions change when it's time to make crucial decisions as a result.
External data may come in different formats and may contain errors or missing values. To use the data effectively in forecasting models, data scientists need to clean and preprocess the data. This involves removing duplicates, correcting errors, filling in missing values, and transforming the data into a format that can be easily integrated into your forecasting models.
Integration with Forecasting Models
Once the data is processed, it needs to be integrated into forecasting models. Various techniques can be used to incorporate external data into forecasting models, such as statistical models, machine learning algorithms, or other forecasting techniques. Experimenting with different models and techniques is crucial to find the best fit for your data and forecasting needs.
Finally, to ensure accuracy and reliability, it is crucial to validate and refine your forecasting models. This involves comparing the forecasted demand with the actual demand and making adjustments as needed. Refining the models over time may be necessary as new external data becomes available or as market conditions change.
Conclusion
This article provides a few examples of the role external data plays in forecasting and outlines the steps needed to initiate the use of these assets in a modeling pipeline when leveraging AI-powered category forecasting projections. By incorporating both internal and external data, businesses can create models that are sensitive to both their operations and the broader market conditions in which they operate.
Discover Visionet's AI-based Forecasting Solution, the ultimate tool for predicting future sales and demand in today's dynamic retail environment. Harnessing the power of internal and external data sources, our cutting-edge system delivers superior accuracy, empowering retailers to make smarter, data-driven decisions. Stay ahead of the competition and unlock a more profitable future with Visionet's easy-to-use, high-performance forecasting solution