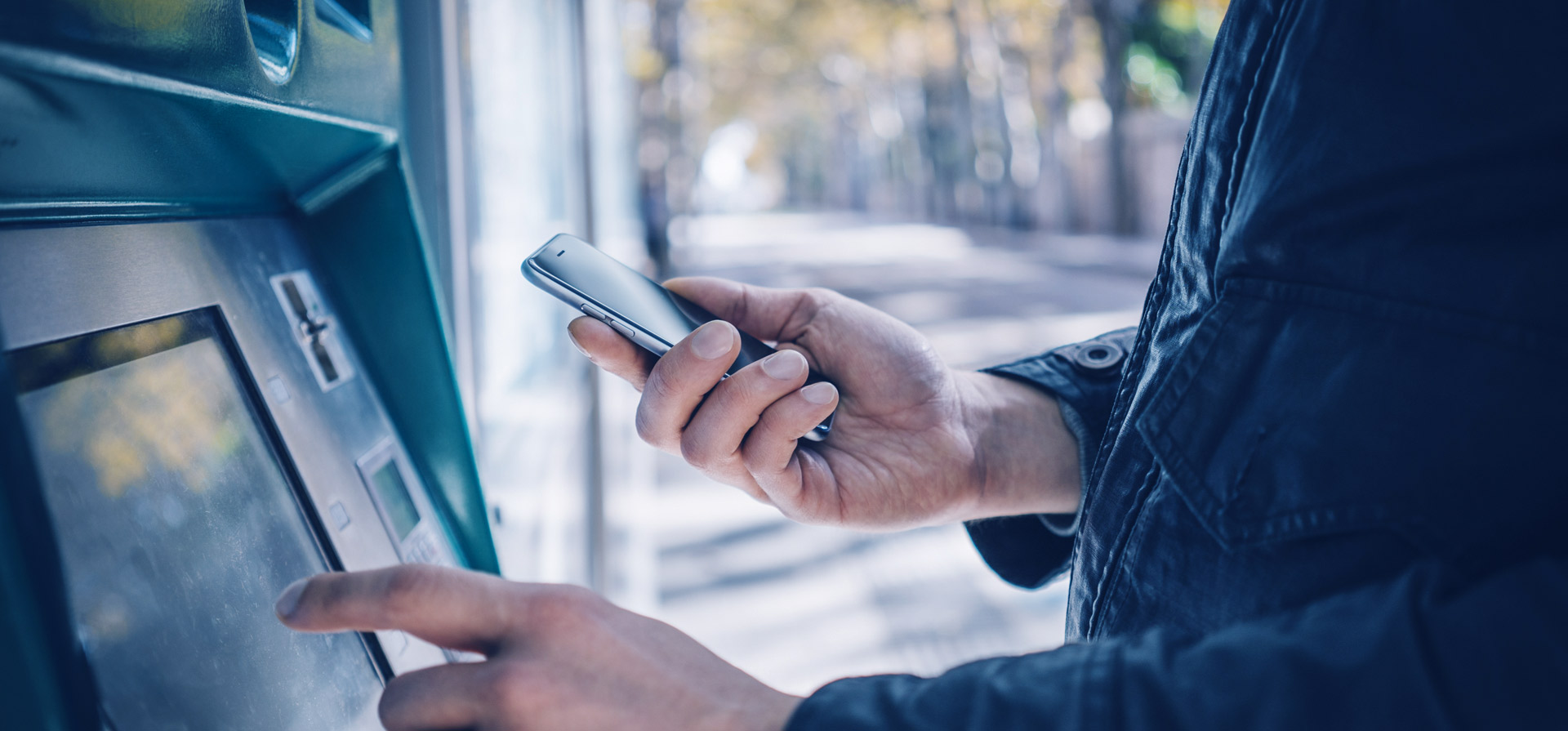
Listen to this blog
Efficiently managing cash in automatic teller machines (ATMs) can reduce financial costs due to unused stocked cash. Predicting cash demand is challenging because of the unpredictability of withdrawals, but profitable because of the large number of machines. Over 3.5 million ATMs are used all over the world.
More banks are shifting their attention to driving greater efficiency in how they manage cash in their ATM networks.Through currency management optimization, banks can avoid falling into the trap of maintaining too much cash and improve profits by mobilizing idle cash. Therefore, it is crucial to develop advanced algorithms to accurately predict cash demand for each ATM. An intelligent cash management system based on cash demand forecasting can then help banks lower operational costs and improve the return on their cash assets.
Using the past to predict the future
Usually, ATM cash management and optimization is done manually, relying on corporate policies and personnel experience. However, financial institutions now have sufficient historical records of ATM transactions and the compute power to analyze these transactions using machine learning. For this reason, humans should hand over the primary responsibility of optimization to machine learning, and instead assume a supervisory role, identifying and addressing exceptions that historical analysis can’t account for.
An efficient ATM cash management system needs a cash demand forecasting model for each ATM. This forecasting model is mostly based on historical cash demand data. Cash withdrawals are subject to trends and generally follow weekly, monthly, and annual cycles. For example, people have a habit of drawing out comparatively large sums of cash at the start of each month. However, the cash demand for every ATM is different, changes over time, and is affected by several factors, including highly mobile users, paydays, holidays, and seasonal demand in specific areas.
Harnessing artificial neural networks
Artificial neural networks (ANNs) are extremely flexible function approximators that are used in machine learning applications such as pattern recognition, classification, and time series forecasting. ANNs map the nonlinear relationships between numerous factors affecting cash withdrawal and cash demand. Once these relationships are identified, the ANN provides a cash demand forecast.
The success of the ANN depends on the configuration of its internal parameters and the quality of the historical data used to train it. ANN configurations can use a different number of association layers, a different number of nodes in their input, association, and output layers, different learning rates, different levels of tolerance for error, and so on. The complexity of ANN configuration requires the expertise of a highly trained data scientist to yield consistently accurate results.
Applied specifically to cash optimization, ANNs allow banks to more accurately estimate the number of bank notes of each currency and denomination required by users of each of their ATMs. These improved estimates help avoid instances of ATMs running out of cash without large amounts of excess cash sitting unused in ATMs. Machine learning can also improve operations by determining optimal replenishment schedules, the best time of day to restock each machine, the ideal delivery routes for servicing multiple ATMs, and given the right data, could even suggest the most profitable locations for future machines.
Conclusion
Cash forecasting is important aspect of effectively managing ATM networks. Cash management solutions make the process more agile and reliable by automatically generating a network-wide plan and making sure each of your machines has enough cash. Since machine learning techniques adapt to changes in demand, investing in machine learning solutions provides long-term ROI. For more information about our innovative machine learning and data science solutions, please contact Visionet Systems.