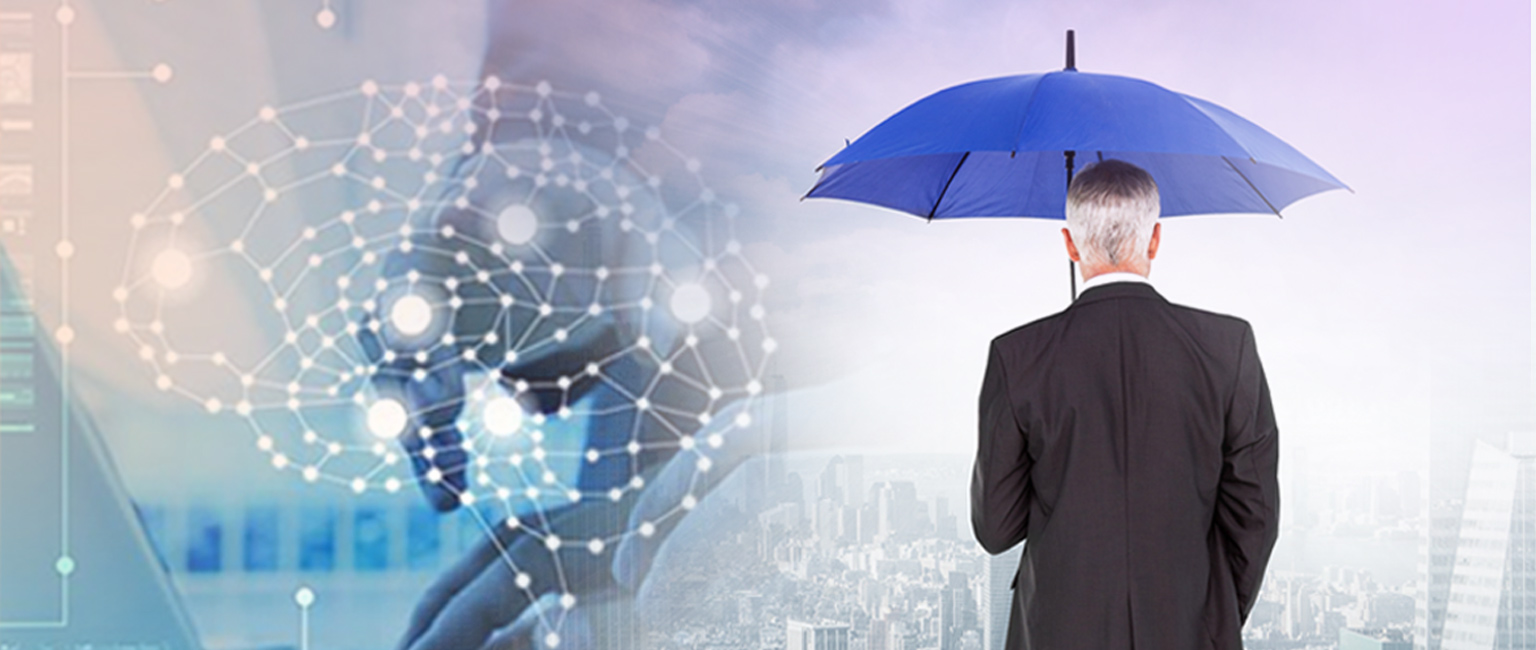
Listen to this blog
The post-COVID era has brought about new challenges in quantification in insurance as companies look towards new approaches and tools to quickly identify emerging patterns in the industry. Shorter time to market for new products, and the timeliness of necessary steps to risk mitigation or prediction will be the key to successful portfolio management.
Artificial Intelligence in the Insurance Industry
Artificial intelligence (AI) is radically transforming many industries, but few industries rely on its foundation – data – as deeply as the insurance industry. Artificial intelligence (AI) in the insurance industry will shape the future of intelligent insurance. As AI becomes more deeply integrated in the industry, insurers are turning to it because of its tremendous benefits across the insurance value chain. One area of the industry that AI is reimagining is target market segmentation.
While the traditional segmentation approach still works, it is less effective in today’s consumer-centric and highly competitive business landscape.
Read more: AI-Powered Big Data Analytics for Insurance is the New Normal
Traditional Approach
Customer segmentation is the building block of any portfolio management. The traditional approach to customer segmentation relies on predefined experience-based variables. There will be substantial slice-and-dice of the selected variables to generate key insights that drive business. The limitation of this approach is that the segment space is limited to business user domain, data available with limited variations and bias towards certain variables, which leads to extensive analysis within a limited scope of work. These segmentations have a major influence on different areas of the insurance domain from underwriting to claims processing. Traditional approach’s capabilities are also limited when using alternative data where machine learning techniques are required to connect the dots.
AI-Driven Customer Segmentation
Insurance companies of today need closed-loop optimized segmentation models with the ability to leverage systematic data exploration methods to view the data in hand, extract valuable insights from new machine-generated data, and correlate data with third-party sources, digital channels, as well as economic indicator overlays.
AI-driven segmentation’s biggest advantage is that it is not biased with business user intuition and is able to include feature sets traditionally not included by conventional wisdom. Machine learning algorithms can leverage existing data elements and devise new feature sets that are able to define customer segments more efficiently. Traditionally, if 50 data elements were used on an average for customer segmentation, machine learning can use more than 1000 data variables with reasonable relationships with the desired target variables (lapse ratio, claim, risk profile, profitability, etc.). These features can be derived by using a combination of existing variables, geo-sensitive third-party data, social media, economic indicators and internet search indexes.
The biggest advantage of AI-driven segmentation over traditional segmentation is the capability to quickly discover changes in customer segments. By incorporating geo-sensitive third-party data and economic indicators, the resultant model can swiftly and effectively identify anomalies and emerging trends.
Key Considerations in AI-Driven Segmentation
Visualization of Customer Segments
AI-driven segmentation for business users is a black box using many complex features derived from underlying data. Artificial intelligence comes with millions of different segments that can be filtered out by confidence levels and other target variables (lapse ratio, claim, risk profile, profitability, income, etc.) to effectively articulate emerging customer segments.
Visualization of Visionet’s Insurance Data Platform showing holistic view of AI-generated customer segments with option to select key data starting points and confidence level of segment strength. The platform enables thorough understanding of segmentation data without getting into the complexity of the model.
Unified Data Platform
Traditional segmentation is normally performed in silos and is limited to departments within specific areas of insurance to achieve desired results within a limited scope, missing out on the big picture. AI-driven segmentation equips the insurance data platform with portfolio-level, customer 360-degree, as well as transaction-level data, unlocking the ability to combine data from third-party and digital sources to create deep actionable insights at a more granular level.
Visionet Insurance Data Platform technology stack
Closing the Loop
No AI or data-driven initiative can succeed without having the complete fulfilment cycle in order to learn and improve. The key elements of successful AI-driven customer segmentation include drill down analysis at the customer level, customer tagging, CRM integration, tracking decisions taken on particular customers, and breaking performance down to quantifiable metrics. AI-driven segmentation model can also be optimized over time which makes it ideal to gain a competitive advantage in a highly disruptive industry.
Read more: From Thought to Bot: The Rise of Commercial AI
Conclusion
The key value of artificial intelligence in insurance industry segmentation is exploring new and hidden dimensions of customers in an unbiased and automated fashion. The challenge is to unlock those key insights generated by AI-driven segmentation without complexities for business users. Visionet’s Insurance Data Platform presents these insights in layers which can enable actionable intelligence and informed decision making for business users.
Get in touch with our experts to know how we can mobilize data to accelerate business growth through Business Intelligence, Data Modelling, and AI-powered advanced analytics.
Author
Chaudhry Sohail
Data Analytic & Growth Leader