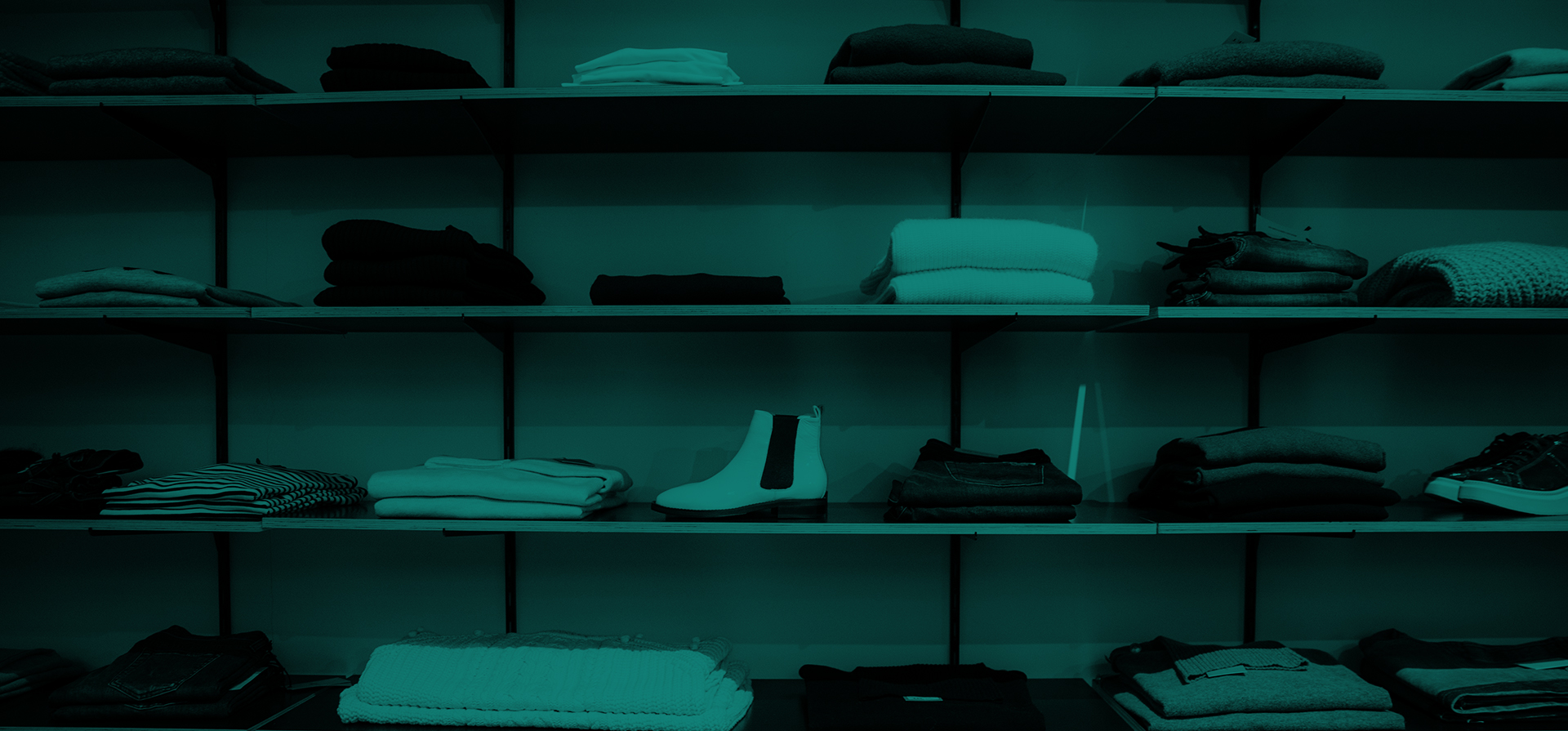
Listen to this blog
As digital services permeate every aspect of the world around us, data has begun to assume a central role. With increasing customer demands, growth expectations, and the need to evolve rapidly, data is an organization’s biggest source of competitive advantage. Real-time insights gathered by data are crucial to support digital initiatives and allow organizations to make better operational choices.
Treating data as core, not an afterthought
Business success depends on timely and accurate data. However, in many organizations, each department (e.g. Marketing, Sales, Merchandising, etc.) generates, analyzes, and manages its own “silo” of information. Unfortunately, these data silos inhibit enterprise-wide collaboration, knowledge sharing, and analysis. Forward-thinking organizations have naturally progressed from narrowly scoped data-driven insights aimed towards a particular need to centrally driven insights based on data collected from across the organization.
A data-centric enterprise treats data collection and analysis as a vital activity instead of a “by the way” consideration. This requires the unification of data silos to create a single, reliable source of truth. A data-centric organization can efficiently apply machine learning and predictive/prescriptive analytics to create impactful sales strategies, optimize shipping schedules, minimize waste during manufacturing, and much more.
Positive outcomes for Marketing, Sales, and Merchandising
Here are just a handful of examples of how adopting a unified enterprise data platform can transform your marketing, sales, and merchandising processes:
Marketing
Equipped with accurate data on who’s buying each of your products, you can perform customer segmentation with a high degree of precision. Your Marketing department can then use this segmentation to maximize ROI by focusing your marketing spend on the most receptive audiences. You’ll also improve engagement and conversion by crafting inbound and outbound marketing campaigns with highly relevant messaging that resonates well with each segment.
Social listening provides a direct window into how your customers behave, what they like, and what they’re most likely to buy. Gathering and using this data to create promotions and incentives is a great way to attract and retain a loyal customer base.
A truly data-centric organization collects data from every aspect of its operations, including customer behavior across all channels. This creates a 360° customer view that you can use to create a highly effective customer journey with personalized messaging across multiple customer touchpoints.
Sales
Your business data can also help you create a winning sales strategy and drive revenue. Using machine learning to analyze your historical sales data is a powerful way to discover the best products to bundle or cross-sell. You’ll also be able to identify the best range, pricing mix, and sales promotions for each product category.
For example, you might find out that your customers often buy popcorn and DVDs on the same visit to your department store. Machine learning can help you find the best bundle price for those two types of products. You can simulate the difference between using budget-brand versus premium-brand popcorn in the bundle, or substituting DVDs for Blu-Ray discs.
Merchandising
How many facings of each product should you have on your shelves? By collecting information about the flow of customer traffic and product offtake within your stores, you can optimize the SKU positions in your plan-o-grams and increase the visibility of product categories. Advanced analytics platforms use demand forecasts, inventory turnover targets, and other performance indicators to determine the right quantities to display for each product.
Enterprise data platforms analyze historical sales data to improve each store’s assortment plan, optimize their product mix, and boost profitability. “Intelligent shelving” techniques” can help you enhance physical merchandising, optimize product placement, and make more efficient use of your floor space.
Aggregate Benefits
Consolidated data form all departments and channels can generate more actionable intelligence than each department’s data silo can on its own. While your Marketing department’s successful campaigns, your Sales team’s pre-season sales strategy, and your Merchandising department’s assortment plans are valuable, their value multiplies when they’re combined.
For example, analyzing historical sales without any knowledge of your marketing activities will lead to inaccurate demand forecasts that don’t take promotional markdowns into account. Similarly, lack of coordination between Merchandising and Marketing will result in inefficient shelf utilization during pop-up sales. Even though you have all the business data you need, the absence of a data-centric approach obscures the insights you need for success.
Conclusion
Consolidating the collection and analysis of business data across all departments and business functions results in lower operating costs, fewer reporting delays, improved business agility, and access to a wider range of valuable insights. You need a reliable and scalable enterprise data platform to enable such a data-centric approach.
For an in-depth exploration of enterprise data platforms, watch the recording of Visionet’s webinar, “A Strategic Necessity: Enterprise Data Platforms”, presented in partnership with the American Apparel & Footwear Association (AAFA).