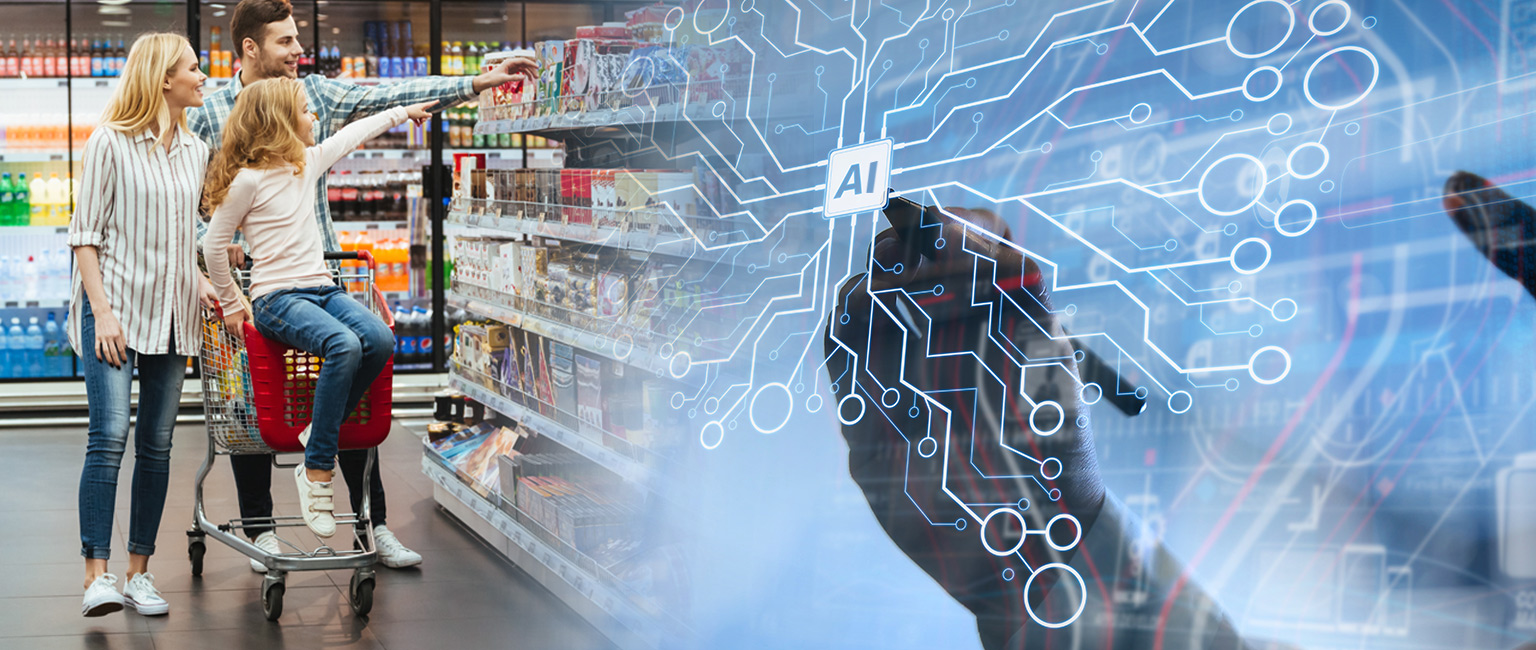
Listen to this blog
Demand forecasting has become a key component in the eCommerce and retail industry. While analysts often employ it manually with the use of ERP solutions to optimize stock levels, increase efficiency and elevate customer experiences, advancements in artificial intelligence have taken demand forecasting to a whole new level.
Here are 4 ways how artificial intelligence is reshaping retail demand forecasting:
1. Data consolidation for retail demand forecasting accuracy
Traditional retail demand forecasting systems typically involve analyzing historical sales data taking into account seasonal variations. However, it is a multi-dimensional problem and is influenced by various factors. AI-powered forecasting systems are capable of using data from multiple sources and derive complex and hidden relationships between them which can be strong predictors of demand. These systems typically provide more accurate forecasts as more and more historical data becomes available, which proves its worth in demand forecasting in the retail industry.
Data sources can be internal, external and contextual.
Read more: Tracking, Reporting and Forecasting Retail needs
Internal Data
A company’s internal data can provide a wealth of information on sales, product characteristics, metadata, marketing and promotional activities, to name a few. Data from marketing and promotional activities is probably the most valuable internal data. Combining the demand forecasting system with historical marketing and promotions data can unearth how demand changes by lowering prices, for instance. It can also tell how much to lower the prices to increase demand and revenue.
External Data
These sources are also important and can include sales data from distributors, POS or market research reports. Businesses selling through distributors can use AI-driven demand forecasting to spot the factors influencing increased demand from different distributors.
Contextual Data
Contextual sources can provide insights on demographic or geographic data and seasonal demand. Demand is always context-specific in every business and can change for a product seasonally. AI models can handle changes in demand on a day-to-day basis and produce better forecasting. As holiday seasons are very society-specific, demographic data can help define more contexts in which sales take place. If a brick-and-mortar store knows the purchasing power of the local population, it can improve the forecasting of specific product categories.
2. Demand anticipation for new products
At its core, demand forecasting is about predicting future sales based on historical sales data of a given product. However, there is no historical data for new products. Comparing characteristics of new products to the attributes of the ones previously sold can produce a valuable demand forecast. An eCommerce fashion retailer can use information about garment characteristics like cut, color, pattern, texture, material, as well as photos of the garments to find similar items that were sold before and assess how those distinctions impacted sales. AI-based systems use the most sophisticated forecasting algorithms to predict demand, the accuracy of which usually ranges from 70% to 90%. These algorithms learn and improve through experience as more historical data is added.
3. Marketing and promotional impact prediction
By analyzing historical data, the AI-driven demand forecasting systems can break the demand into ‘real’ demand and the ‘promotional’ effect. Retailers that run frequent promotions can find this system to be extremely helpful. They can test how a given promotion will affect sales and therefore pick the optimal price point. By implementing techniques like Market Basket Analysis, such systems are also capable of modelling complex relationships between inventory items that might not be possible to identify otherwise. These can also provide analytical insights into customer behavior which can be used by decision makers to plan and implement targeted marketing and other promotional campaigns. Intelligent demand forecasting systems are also capable of simulating different scenarios, such as, gauging how a new clothing line will be received by the existing customer base if it is launched in the first quarter of the coming fiscal year. This can aid the retailers in planning new product launches.
4. Monitoring and analysis of demand irregularities
Demand forecasting systems can also help monitor current activities, not just forecast the future. In eCommerce, irregularities can arise due to technical glitches or natural spikes in demand. AI can help detect these anomalies for immediate action by integrating multiple streams of data such as real-time activity on a website. For instance, if a retailer observes a huge spike in face mask orders, it can quickly place a substantial order with its distributor before the others do. Similarly, an unusual order placed for hundreds of items by someone on a fake address can be an indication of a fake/fraudulent customer order. An intelligent retail demand forecasting system can send an alert to verify details from this customer before the order is processed.
Conclusion
As consumer buying behavior continues to change, demand forecasting in retail industry is evolving. Retailers have access to better information and technology to drive better forecasts. A demand forecasting system equipped with cutting-edge AI capabilities enables optimized inventory levels, highly effective marketing and promotions, as well as fewer man-hours spent on forecasting. It also enables flexibility to adapt to changes in the existing processes.
Visionet’s AI-powered inventory planning solution allows retailers to leverage all the data sources at hand to see the bigger picture of their business and strategize accordingly. Get in touch with our experts to learn more.